Unintended Consequences: The Perils of Publication and Citation Bias
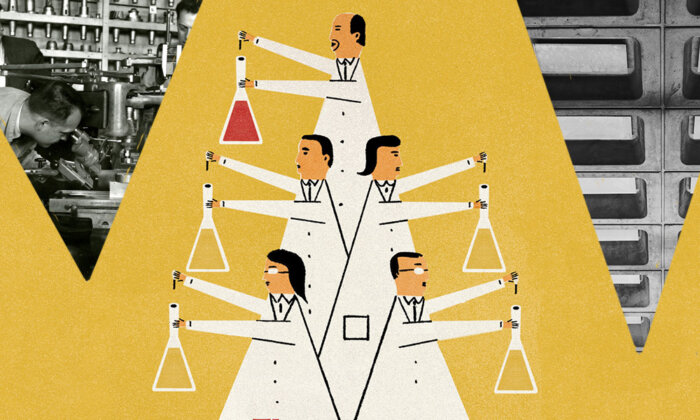
When President Donald Trump promoted hydroxychloroquine as a cure for Covid-19, Dr. Anthony Fauci, a leading White House advisor on the novel coronavirus, didn’t hesitate to contradict him. Fauci declared that all the “valid” scientific data showed that the drug was, in fact, ineffective.
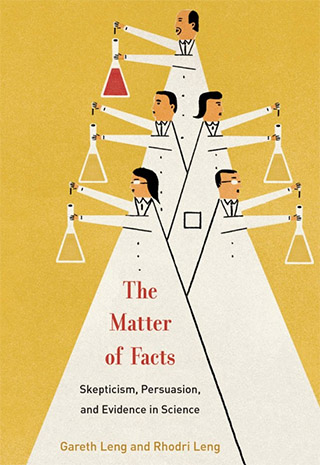
What Trump had done wasn’t particularly unusual — he ‘cherry-picked’ evidence to support a claim that he had prematurely committed himself to, without recognizing the weakness of that evidence and without acknowledging the strength and abundance of contrary evidence.
Fauci, in contradicting Trump, was observing what many scientists would recognize as a moral imperative in science — to look at all the relevant evidence, and to weigh it dispassionately, using objective criteria. While scientists will readily acknowledge that this is what they should be doing, they can fall short of this standard in their own work.
Recently, some have begun inspecting the quality of evidence in the scientific literature, how that evidence is used, and how it spreads and shapes opinion in the scientific community. Of particular concern to scientists — and increasingly, a science-literate public — are dissemination biases. The existence of these is provoking concern that the integrity of Science is being undermined and that myths or half-truths can spread rapidly through the scientific literature.
Publication Bias
The best known of these biases is publication bias, which arises from scientists preferentially publishing experiments with statistically significant (“positive”) outcomes. In 1979, psychologist Robert Rosenthal coined this term the “file drawer problem,” the problem being that researchers are keeping unpublished results that don’t support their hypotheses tucked away and out of sight.
By the late 1980s, publication bias had become known for its detrimental impact on medical advice.
Rosenthal feared that the failure to publish findings that either failed to reach statistical significance or found an effect opposite to that predicted would skew the scientific literature in favor of positive outcomes. And this, in turn, might lead to the overestimation of the benefits of particular treatments, flawed scientific advice, and wasted research.
By the late 1980s, publication bias had become known for its detrimental impact on medical advice. In 1987, Kay Dickersin and colleagues asked 318 authors of randomized controlled trials whether they had been involved in any trial that had gone unpublished. The 156 authors who responded reported the existence of 271 unpublished trials — about 26 percent of all those they had been involved in. Of the unpublished trials, 178 had been completed and only 14 percent of these supported the theory under investigation compared to 55 percent of the published trials. It seemed that authors just didn’t bother to write up negative trial results and submit them for publication. Since then, different forms of publication bias have been identified, including:
- Time-lag bias, where trials with impressive positive results (a large effect size, statistical significance) are published more quickly than trials with negative or equivocal results.
- Outcome reporting bias, reporting only statistically significant results or results that favor a particular claim while other outcomes have been measured but not reported.
- Location bias, publishing nonsignificant, equivocal, or unsupportive findings in journals of lesser prestige, while studies reporting positive, statistically significant findings tend to be submitted to better-known journals.
This problem has not gone away. In 2010, the U.S. National Institute for Health Research conducted a systematic study of health-care intervention studies. It found that studies with significant or positive results were more likely to be published than those with nonsignificant or negative results and tended to be published sooner. Published studies tended to report a greater treatment effect than unpublished studies, and this bias affected consensus conclusions of systematic reviews.
In 2010, the NIH found that studies with significant or positive results were more likely to be published than those with nonsignificant or negative results and tended to be published sooner.
In 2015, Michal Kicinski and colleagues examined 1,106 meta-analyses published by the Cochrane Collaboration on the efficacy or safety of particular treatments. For meta-analyses that focused on efficacy, positive, significant trials were more likely to be included in the meta-analyses than other trials. Conversely, for meta-analyses that focused on safety, “Results providing no evidence of adverse effects had on average a 78 percent higher probability to enter the meta-analysis sample than statistically significant results demonstrating that adverse effects existed.”
These were disquieting findings. Cochrane reviews are supposed to be gold standard in the biomedical area, but even here there was bias. The explanation might be either that (1) trials producing nonsignificant, equivocal, or unsupportive findings are failing to be published, or (2) they are being published but are ignored by meta-analyses.
Citation Bias
In the 1980s, researchers noted that studies reporting positive, statistically significant findings were cited more often than studies with nonsignificant or negative findings. The first systematic study of this was Peter Gøtzsche’s 1987 study of clinical trials of anti-inflammatory drugs in the treatment of rheumatoid arthritis. Gøtzsche looked at how the authors had referenced previous trials of the same drug. He searched the literature to find all published trials and classified each paper by whether their authors had interpreted the outcome with the drug as “positive” or “not-positive.” He then looked for evidence of bias in the citations. Positive bias was judged to have occurred if the reference list disproportionately cited trials with positive outcomes. Of 76 papers in which such bias could potentially occur, 44 showed a positive bias. Many authors had preferentially cited evidence that had shown a positive outcome for the drug that they were testing.
In 1992, Uffe Ravnskov looked at how trials of dietary interventions for coronary heart disease were cited. Trials that supported their effectiveness were cited, on average, 40 times per year, Ravnskov found, but unsupportive trials were cited just seven times per year. How often any particular trial was cited was not correlated with its size, nor with the quality of the journal that it was published in. Eight supportive trials were published in major journals and these had been cited on average of 61 times per year; 10 unsupportive trials in similar journals had been cited just eight times per year. “The preventive effect of such treatment,” Ravnskov concluded, “has been exaggerated by a tendency in trial reports, reviews, and other papers to cite supportive results only.”
The decisions of individual scientists to cite only certain papers can have unanticipated consequences that ripple through the literature, shaping what evidence other scientists choose to cite.
Citation bias, too, is now a well-documented phenomenon. In 2012, Anne-Sophie Jannot and a team examined 242 meta-analyses published in the Cochrane Database of Systematic Reviews between January and March 2010, covering diverse research focuses, including cardiovascular disease, infectious disease, and psychiatry. The 242 meta-analyses had referenced 470 unique trials. Trials with statistically significant results for the primary outcome accumulated, on average, more than twice as many citations as trials that did not show a statistically significant primary outcome.
In 2017, Bram Duyx and colleagues reviewed 52 studies of citation bias — 38 on bias in the biomedical literature, seven in the social sciences, six in the natural sciences, and one with multiple focuses. “Our meta-analyses show that positive articles are cited about two times more often than negative ones,” they reported. “Our results suggest that citations are mostly based on the conclusion that authors draw rather than the underlying data.”
Compared to publication bias, however, citation bias received comparatively little scholarly attention until a 2009 paper by neurologist Steven Greenberg sent shockwaves through the biomedical community.
Greenberg had become interested in whether a claim that he had seen regularly in scientific papers was actually supported by the available evidence. It was widely ‘known’ that a particular protein, β-amyloid, was abnormally present in the muscle fibers of patients with inclusion body myositis, a muscle-wasting disease. This claim had important implications for treatment, and Greenberg had seen it repeated in at least 200 papers that had given the impression that this was a “fact.” He wanted to find the evidence for it.
But Greenberg could find only 12 papers that had investigated the claim directly. Of these, six supported it, but six did not. On his reading, there were major technical weaknesses with the supportive evidence. Worryingly, the first four supportive papers all came from the same laboratory, and two of these “probably reported mostly the same data without citing each other.”
Greenberg wanted to understand how this claim, which to him appeared questionable, had become an apparent “fact.” The first 10 of the primary studies were all published between 1992 and 1995, and he looked at how these were cited in the years until 2007. He found 242 papers that discussed the β-amyloid claim. These contained 214 citations to the early primary studies. But 94 percent of these were to the four supportive studies, and just six percent to the six unsupportive studies. The literature was overwhelmingly citing supportive evidence while neglecting unsupportive evidence.
So, how did this happen? Greenberg analyzed how the trial evidence might have spread from paper to paper by tracing the citation links between papers. With this, he showed that review papers had played a major role in directing scientists to the evidence. Knowledge about β-amyloid derived from four reviews: 95 percent of all paths — chains of citation stringing together papers — to the original primary data went through these review papers. These reviews cited the four supportive primary papers but none of the unsupportive studies — they had funneled attention to studies that supported the β-amyloid claim.
Greenberg had demonstrated that citation bias can lead to important distortions in scientific understanding. The decisions of individual scientists to cite only certain papers can have unanticipated consequences that ripple through the literature, shaping what evidence other scientists choose to cite.
But the citation problems don’t end there.
Citation Distortion
In 1980, Jane Porter and Hershel Jick published a five-sentence letter, “Addiction Rare in Patients Treated with Narcotics,” in the New England Journal of Medicine. They had sifted through the records of 11,882 patients who had been prescribed at least one narcotic and had found only four cases of addiction:
“We conclude,” they asserted, “that despite widespread use of narcotic drugs in hospitals, the development of addiction is rare in medical patients with no history of addiction.”
Pieter Kroonenberg, a Dutch statistician, discovered a nonexistent paper that had been cited more than 400 times.
But in 2017, in another letter published in the New England Journal of Medicine, Pamela Leung and colleagues reported that Porter and Jick’s letter had been cited in 608 papers between 1981 and 2017. They read each of these to see how it was cited; 439 of them (72 percent) had used it as evidence that, in patients treated with opioids, addiction is rare. Importantly, 491 of the citing papers failed to report that the letter had described the experience of patients that had been hospitalized; that is, patients in a well-controlled, safe setting under constant close supervision. Leung et al. concluded:
A five-sentence letter published in the Journal in 1980 was heavily and uncritically cited as evidence that addiction was rare with long-term opioid therapy. We believe that this citation pattern contributed to the North American opioid crisis by helping to shape a narrative that allayed prescribers’ concerns about the risk of addiction associated with long-term opioid therapy. Our findings highlight the potential consequences of inaccurate citation and underscore the need for diligence when citing previously published studies.
Here, the meaning of a study was subverted through chains of citation.
In 2010, Andreas Stang published a critique of the Newcastle–Ottawa scale, a scale used in meta-analyses to assess the quality of observational studies. He came to a bluntly critical conclusion:
The current version appears to be inacceptable for the quality ranking of both case-control studies and cohort studies in meta-analyses. The use of this score in evidence-based reviews and meta-analyses may produce highly arbitrary results.
Eight years later, Stang noted that his 2010 paper had received more than 1,000 citations — but virtually all of them were referencing it incorrectly — as though it supported the use of the Newcastle-Ottowa scale! To show the scale of this misquotation, Stang and colleagues read citing systematic reviews. In 94 of the 96 reviews they identified, Stang’s critique was cited in a manner that suggested it was supportive of NOS.
By September 2020, Stang’s critique of the scale had been cited 5,088 times; but no more accurately than before. His attempt to combat the spread of this misquotation appears to have fallen on deaf ears; his follow-up analysis has been cited just five times.
But how common are such citation errors?
Hannah Jergas and Christopher Baethge reviewed 27 studies on the accuracy of citations in the biomedical literature, identifying (i) major errors that seriously misrepresented or had no resemblance to the referenced paper; (ii) minor errors that contained factual inaccuracies. They concluded that, overall, about one in four references are “wrong or problematic,” while one in eight or nine are “seriously incorrect.”
Sometimes references are simply copied from one paper to another. It is hard to know how common this is, but Pieter Kroonenberg, a Dutch statistician, discovered a nonexistent paper that had been cited more than 400 times. The phantom paper was cited as:
Van der Geer, J., Hanraads, J. A. J., Lupton, R. A. 2010. The art of writing a scientific article. J Sci. Commun. 163 (2) 51–59.
This originated as a hypothetical example given in a style guide used by Elsevier to illustrate how to reference in particular journals. We confirmed, by a search in the Web of Science, that it had been cited more than 480 times by 2019. Most of these came from abstracts in conference proceedings, and it seems likely that many authors had misunderstood that this was an example of how to cite, not an example of something that should be cited. But the reference also appeared in 79 journal papers. Of these, 13 were connected together through references, and in these, it was bizarrely used to support the claim that a compound called rutin could dilute the blood, reduce capillary permeability and lower blood pressure. Here, it seems, this reference was simply being copied from one paper to another.
Underutilization of Evidence
To understand the extent to which the available evidence is utilized, researchers Karen Robinson and Steven Goodman examined how often clinical trials were reported by the authors of later, similar trials. They identified 1,523 trials and tracked how these had cited others on the same topic. Only about a quarter of the relevant trials were cited, which also constituted only about a quarter of the subjects enrolled in relevant trials. Strangely, a median of two trials were cited regardless of how many had been conducted; seemingly, while scientists may see further by standing on the shoulders of those who have gone before, two shoulders are enough, however many are available.
The authors concluded, “Potential implications include ethically unjustifiable trials, wasted resources, incorrect conclusions, and unnecessary risks for trial participants.”
Implications
The system of scientific communication appears to be more fragile than was once believed. It is impacted by researchers’ decisions of what to publish and what to cite. Decisions made by individuals — individuals with particular research goals, expertise, finite memories, and an innate propensity for error — can affect what others believe in ways we still do not quite understand.
If evidence goes unpublished, it may rob others of the opportunity to critique a position. If citation bias is common, then scientists are likely to be basing their understanding on only a partial selection of the evidence. But while we may point to the waste in research time and funding if existing evidence is not fully utilized, and the inherent problems for the validity of scientific claims, the solution is not obvious. While these problems seem to be increasing, this might, however, simply reflect greater recognition of them. That greater recognition may itself drive self-correction in the behavior of scientists.
Gareth Leng is Professor of Experimental Physiology at the University of Edinburgh, the author of “The Heart of the Brain: The Hypothalamus and Its Hormones” and co-author (with Rhodri Ivor Leng) of “The Matter of Facts: Skepticism, Persuasion, and Evidence in Science,” from which this article is adapted.
Rhodri Ivor Leng, an ESRC Fellow at the University of Edinburgh, specializes in citation network analysis.