The Myth of Objective Data
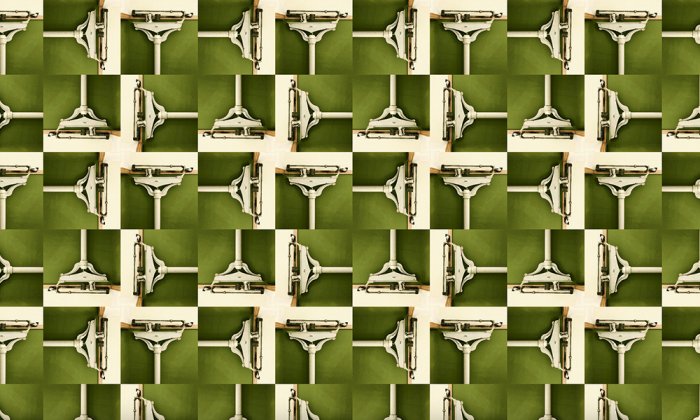
The notion that human judgment pollutes scientific attempts to understand natural phenomena as they really are may seem like a stable and uncontroversial value. However, as Lorraine Daston and Peter Galison have established, objectivity is a fairly recent historical development.
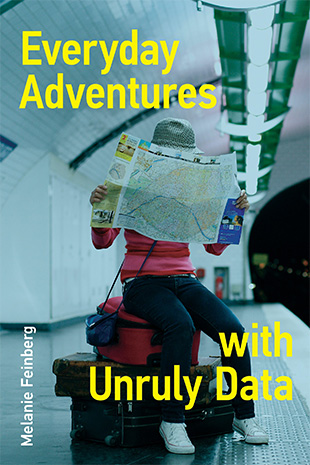
In Daston and Galison’s account, which focuses on scientific visualization, objectivity arose in the 19th century, congruent with the development of photography. Before photography, scientific illustration attempted to portray an ideal exemplar rather than an actually existing specimen. In other words, instead of drawing a realistic portrait of an individual fruit fly — which has unique, idiosyncratic characteristics — an 18th-century scientific illustrator drew an ideal fruit fly. This ideal representation would better portray average fruit fly characteristics, even as no actual fruit fly is ever perfectly average.
With the advent of photography, drawings of ideal types began to lose favor. The machinic eye of the lens was seen as enabling nature to speak for itself, providing access to a truer, more objective reality than the human eye of the illustrator. Daston and Galison emphasize, however, that this initial confidence in the pure eye of the machine was swiftly undermined. Scientists soon realized that photographic devices introduce their own distortions into the images that they produce, and that no eye provides an unmediated view onto nature. From the perspective of scientific visualization, the idea that machines allow us to see true has long been outmoded. In everyday discourse, however, there is a continuing tendency to characterize the objective as that which speaks for itself without the interference of human perception, interpretation, judgment, and so on.
The idea that machines allow us to see true has long been outmoded.
This everyday definition of objectivity particularly affects our understanding of data collection. If in our daily lives we tend to overlook the diverse, situationally textured sense-making actions that information seekers, conversation listeners, and other recipients of communicative acts perform to make automated information systems function, we are even less likely to acknowledge and value the interpretive work of data collectors, even as these actions create the conditions of possibility upon which data analysis can operate.
Our propensity to lose track of the diverse set of interpretive judgments packed into every instance of data collection, and accordingly to diminish the socially situated conditions in which data is created, extends even where data collection appears tightly controlled. Indeed, the interpretive flexibility that pervades data collection has been especially well described in the sciences. Scholars have meticulously documented the sociotechnical processes by which the context of observation is variously assumed, accounted for, forgotten, and reconstructed in the collection, aggregation, and use of scientific data.
To summarize what such studies show, here’s a brief scenario. Let’s imagine that we’re a team of scientific researchers conducting a Movement Census project. To determine how much the residents in our area move every day, we’re collecting step counts and distance traveled from a diverse set of smartphone users over a period of two weeks. We know that different phones produce different results, so we make sure to document the hardware and software for each study participant. We also know that gaits vary, so we instruct participants to select from three gait styles: smooth, bouncy, and semi-bouncy. Subsequently, we develop a normalization function to equate data for different devices and gaits. Our function performs pretty well: It can account for 80 percent of the variance between phones. We only have resources to test our function on three popular models of Android phones, but the majority of smartphone users have Android phones. Of course, we’ll summarize these limitations in any academic publication that arises from our analysis. We’re responsible scientists.
Over time, however, we disregard our pledge. We gradually forget that our attempt to account for variation between devices and gaits was only partial, not complete. Moreover, we do not fully comprehend the particularities and qualifications that inhere within our dataset. It’s entirely likely, for instance, that some participants had difficulty selecting a single gait style (bouncy or semi-bouncy?) but we, the researchers, didn’t provide a way to select multiple styles or to indicate uncertainty in a selection. Furthermore, our ideas about gait didn’t account for people with physical disabilities or infirmities, who might move differently or use different kinds of prosthetics or supports. Indeed, I could go on and on about the tremendous array of decisions that our Movement Census team made in shaping this very particular dataset, including, of course, the initial idea that step counts are a good proxy for movement. In summary, the quantitative data of step counts arises from a complex and intricate array of interpretive decisions, from the way that we designed our study to the individual actions of the contributing participants. Empirical studies of science invariably show similar conclusions.
The Movement Census scenario represents typical practice, not bad science. The problem, if there is one, does not lie with sloppy data collectors; it lies with our continued reliance on two-cultures dichotomies, in which objectivity and subjectivity can be neatly separated and human messiness can somehow be avoided in data collection performed by humans (or with automated devices created by humans). When we imagine that datasets of properties like step counts speak for themselves, we negate the responsibility we hold for determining which properties will be expressed as data, in what form, and with what parameters.
Nonetheless, despite the undeniably consistent picture that we see across studies of scientific data collection, the desire to remove the human from the data in order to enhance objectivity remains very strong. Invariably, it seems like the ethical move.
Despite the undeniably consistent picture that we see across studies of scientific data collection, the desire to remove the human from the data in order to enhance objectivity remains very strong.
Changing a culture is a major undertaking, and a data culture is no exception. But thinking of these issues as cultural in the first place can help to open the imagination. When I teach information organization to master’s students, the first project that I set them is to design a descriptive schema: a set of specifications for generating data about some group of things. They can choose to describe whatever they want — coffee beans, computer programming languages, or mythological beasts; it doesn’t matter. Initially, everyone thinks this project is beneath their capabilities: a rote task. When I explain that the whole point is to treat the description of landscape paintings or laptop computers as an open design problem rather than a reification of convention, my students are dubious. How else would we describe science fiction movies if not in the way that Netflix describes them? How else would we describe pain medication if not in the way that pharmacies describe it?
The students think that data is a matter of describing things as they are, and that there is no art to it and certainly no fashion. They very much want to let things speak for themselves when they approach a project like designing a schema to describe a set of things. What my students paradoxically fail to realize, in their zeal to be responsible, is that describing things by certain characteristics rather than others merely because those characteristics are countable is a profoundly subjective decision.
I remember vividly one especially conscientious student who designed a schema for describing socks. To keep her data as objective as possible, she specified only quantitatively measurable attributes, such as thickness in millimeters, circumference of the ankle opening, and precise composition of materials. She avoided anything that had the appearance of human judgment, such as what the socks might feel like on human skin, what outfits they might complement, or their stylishness. But was her data objective? Not at all. The circumference of the ankle opening? That’s one of the most subjective data elements I could have possibly imagined. What a useless bit of data! It was selected solely because the data creator had a personal preference for the appearance of objectivity. When we view objectivity and subjectivity as opposites rather than complements, this is the kind of trap we find ourselves falling into.
This two-cultures thinking, moreover, distorts the empirical realities of data collection, the challenging work of forcing unruly phenomena to speak in clean, distinct, ideally quantitative phrases. It is likely, for instance, that the designer of the sock schema considered the actual measuring of a sock’s ankle opening to be unskilled drudgery, something anyone could do. But even the bare mechanics of measuring a floppy circle are tricky. And there are ontological complexities also. Are we measuring socks as unique material items (for every sock in the world, new or worn, a measurement) or are we measuring socks as a class of equivalent copies (one measurement for a set of equivalent socks, e.g., a particular brand and type)? Even sock measuring is not a mindless task.
“This project is much harder than you think,” I caution my students before they begin designing their schemas. “Most of you will be in despair at some point. In fact, this is how you will know if you are proceeding in the right way: if you suddenly realize that you have no idea what you are doing.” Everyone laughs. They humor me. After all, I am the one with the power; I am grading them.
If I am lucky, though, it really does happen as I theatrically foretell. Everyone feels despair. This despair is a little bit magical. I treasure it! It’s the despair of the unknown possibility. This despair helps my students recognize an apparently banal assignment as a real design situation. It teaches them that data is created, not found; and that creating it well demands humanity, rather than objectivity.
Melanie Feinberg is Associate Professor in the School of Information and Library Science at the University of North Carolina at Chapel Hill. She is the author of “Everyday Adventures With Unruly Data,” from which this article is adapted.